NeRFInvertor: High Fidelity NeRF-GAN Inversion for
Single-shot Real Image Animation
Abstract
Nerf-based Generative models have shown impressive capacity in generating high-quality images with consistent 3D geometry. Despite successful synthesis of fake identity images randomly sampled from latent space, adopting these models for generating face images of real subjects is still a challenging task due to its so-called inversion issue. In this paper, we propose a universal method to surgically fine-tune these NeRF-GAN models in order to achieve high-fidelity animation of real subjects only by a single image. Given the optimized latent code for an out-of-domain real image, we employ 2D loss functions on the rendered image to reduce the identity gap. Furthermore, our method leverages explicit and implicit 3D regularizations using the in-domain neighborhood samples around the optimized latent code to remove geometrical and visual artifacts. Our experiments confirm the effectiveness of our method in realistic, high-fidelity, and 3D consistent animation of real faces on multiple NeRF-GAN models across different datasets.
Method overview
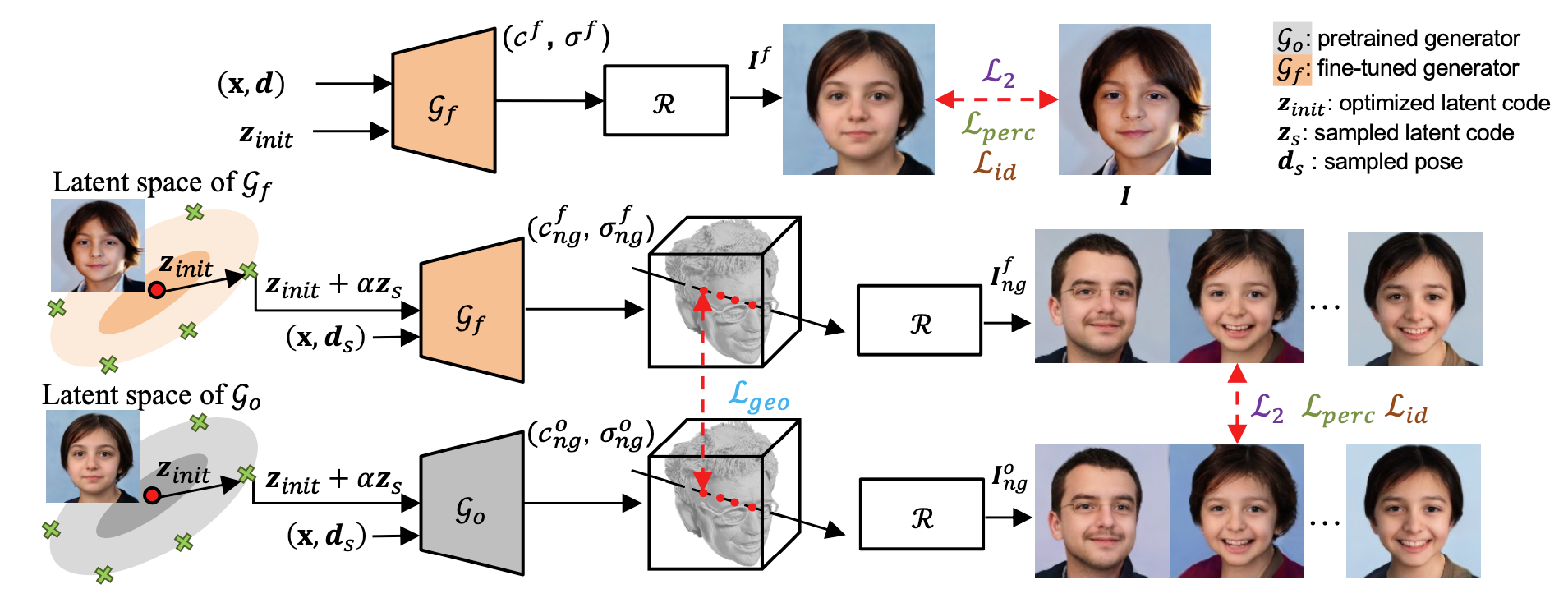
Framework of NeRFInvertor. Given the optimized latent code z_init, we fine-tune the generator and first apply image space supervision to push the generated image to match the input image in the original view d. To augment the NeRF-GAN manifold without worrying about visual artifacts in novel views, we then leverage the surrounding samples of the optimized latent code to regularize the realism and fidelity of the novel view and expression synthesis.
Virtual talking faces driven by real videos
Comparison with Inversion Methods
Comparison with Single-shot NeRF Methods
NeRFInvertor on GRAM (256*256)
NeRFInvertor on EG3D (512*512)
Ethics and responsible AI considerations
This work achieves 3D-consistent and ID-preserving animation (i.e. novel views and expressions) of real subjects given only a single image. It is not intended to create content that is used to mislead or deceive. However, like other related face image generation techniques, it could still potentially be misused for impersonating humans. We condemn any behavior to create misleading or harmful contents of real person, and are interested in applying this technology for advancing 3D- and video-based forgery detection. Currently, the images generated by this method contain visual artifacts that can be easily identified. The method is data driven, and the performance is affected by the biases in the training data. One should be careful about the data collection process and ensure unbiased distrubitions of race, gender, age, among others.
Citation
@inproceedings{yin2023nerfinvertor,
title={NeRFInvertor: High Fidelity NeRF-GAN Inversion for Single-shot Real Image Animation},
author={Yin, Yu and Ghasedi, Kamran and Wu, HsiangTao and Yang, Jiaolong and Tong, Xin and Fu, Yun},
booktitle={Proceedings of the IEEE/CVF Conference on Computer Vision and Pattern Recognition},
pages={8539--8548},
year={2023}
}